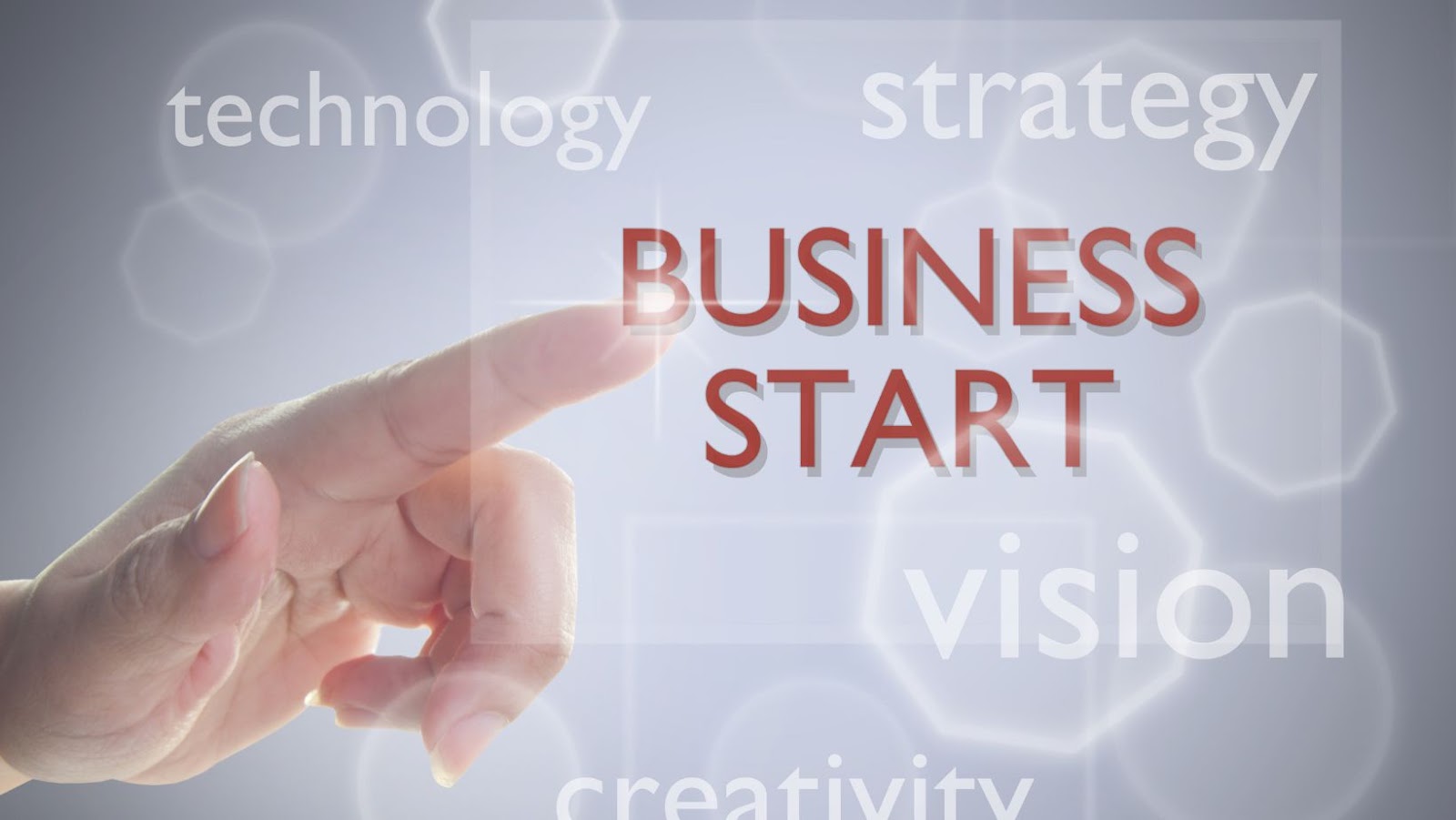
Wallaroo Labs, an innovative machine learning (ML) platform provider, recently announced their Series A funding round of $25 million, led by Microsoft’s venture capital arm M12.
This funding will accelerate the Wallaroo Labs MLOps platform’s development and enable Wallaroo to extend its reach from the cloud to the edge.
This announcement raises the question: What does this mean for the future of MLOps? Let’s explore this question.
Overview of MLOps
MLOps, or “Machine Learning Operations,” is a term used to describe the practice of automating deployment and management of production machine learning (ML) systems. It’s becoming increasingly necessary as organisations use ML in their operations more and more due to its ability to produce better and more accurate insights faster than manual processes. MLOps is meant to make ML deployment, monitoring and updating easier and less time-consuming for developers.
At its core, MLOps aims to provide a continuous deployment process for ML models, allowing organisations to quickly deploy them with minimal effort or disruption. This is done through automation processes that integrate software development best practices such as version control management, automated testing, CI/CD pipelines, and configuration management tools. Additionally, containerization techniques can make it easier for models created in different frameworks or platforms to communicate with each other.
Adopting an MLOps infrastructure gives developers the freedom to focus on building their models instead of worrying about deployment processes while ensuring they are deployed consistently in a way that safeguards their performance in production environments. Furthermore, as organisations become more comfortable with MLOps, they can develop fully automated deployable solutions that will help bring new complex ideas into action quickly and easily. This will open up huge opportunities within data science teams as it enables them to develop fully operational products faster than ever!
Wallaroo Labs raises $25M Series A from Microsoft’s M12 to help with MLOps from cloud-to-edge
Wallaroo Labs is a San Francisco-based start-up that has quickly become a leader in MLOps. Their focus on cloud-native architectures, AI-powered automation and data science continuous integration, has made the product appealing to enterprise customers and venture capitalists alike. Wallaroo’s recent $25M Series A from Microsoft’s M12 venture fund is an exciting sign of confidence in the future of MLOps.
MLOps is the process of effectively managing machine learning models within the software development life cycle and has gained significant traction in recent years due to the proliferation of sophisticated machine learning models deployed in production environments. Combining automating model management operations with integrated data science Continuous Integration/Continuous Delivery (CI/CD) pipelines offers unprecedented efficiency and reliability for mission-critical machine learning applications.
By leveraging Wallaroo’s proprietary devops platform and its integrations with popular cloud platforms like AWS, Azure and Google Cloud, organisations can significantly speed up their ML initiatives while simultaneously reducing operational costs. Additionally, Wallaroo continues to expand its capabilities through partnerships with leading providers such as NVIDIA which provides specialised hardware acceleration for more complex AI tasks.
The round from M12 will further strengthen Wallaroo’s already formidable suite of products, enabling organisations large and small to efficiently manage, test, deploy and monitor their machine learning models at scale. This funding also serves as a major validation for MLOps as an incredibly valuable tool for businesses looking to gain competitive advantage by quickly developing new AI technologies while keeping operational costs low.
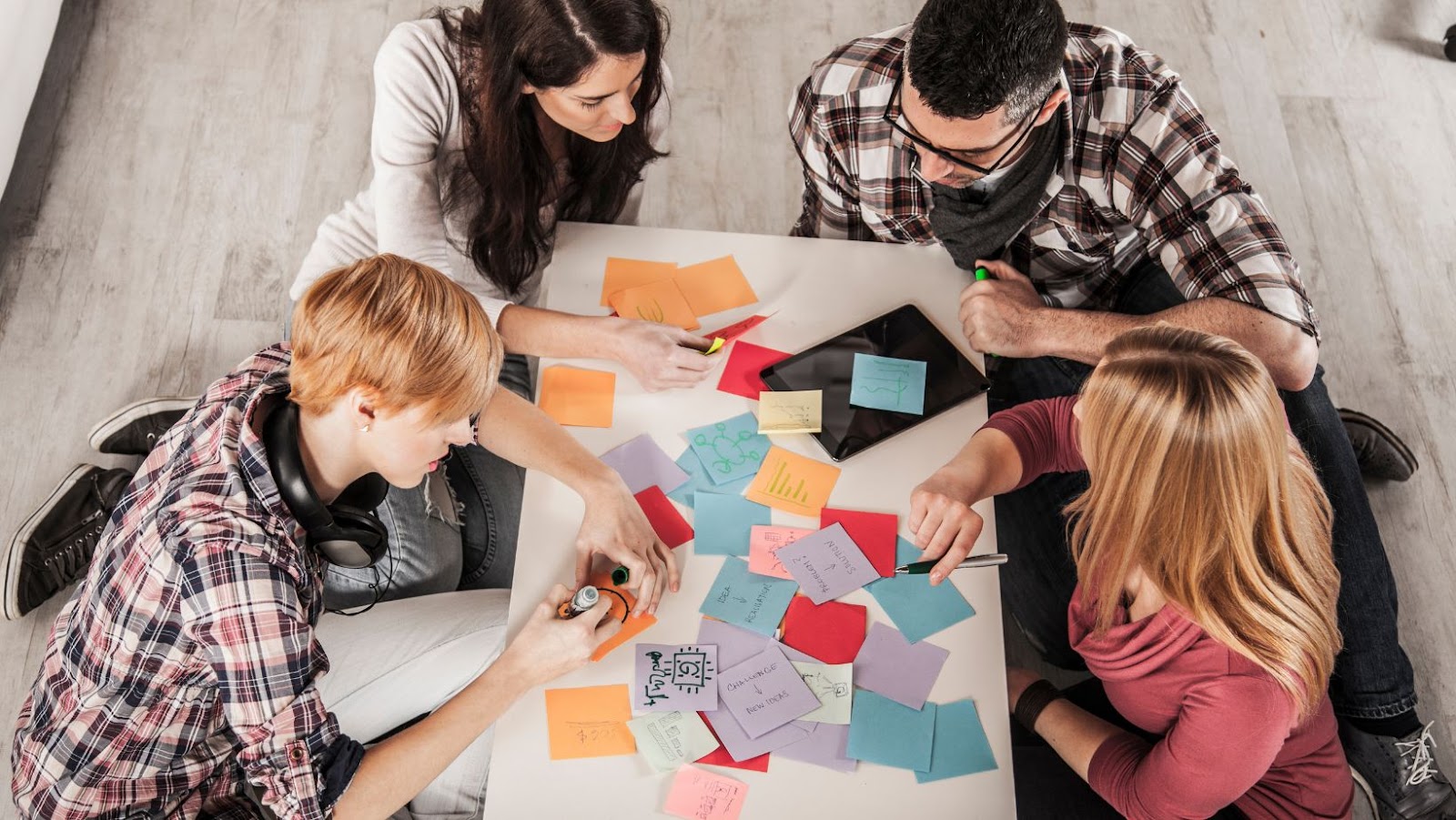
What MLOps Means for the Future
The $25M Series A funding from Microsoft’s M12 for Wallaroo Labs signals a new level of focus on the growing importance of MLOps—or Machine Learning Operations. MLOps is a combination of automation tools and practices that enable the efficient and effective delivery of ML-driven product features. This type of funding indicates the value that Wallaroo Labs can bring to organisations looking to optimise their ML delivery process.
However, what does this mean for the future of MLOps? Let’s take a closer look.
Cloud-to-Edge MLOps
Cloud-to-Edge MLOps, sometimes called Machine Learning on the Edge (Pearling) is a powerful way to move information from the cloud to devices in remote locations. MLOps provides users with a way of connecting machines in physical locations such as factories and warehouses to machines in the cloud. This form of deployment enables analysis and prediction of data generated from geographically distributed sources. In addition, cloud-to-Edge MLOps allows users to improve the accuracy and reliability of their models and data analysis by providing access to more resources than possible with onsite or standalone installations.
Using this form of machine learning, organisations can deploy models and algorithms at predetermined intervals for real-time predictions across the enterprise network. Cloud-to-Edge MLOps helps organisations bridge existing infrastructure investments due to its ability to easily deploy models across multiple clouds or cross cloud environments, easing integration with existing systems. In addition, by leveraging sophisticated monitoring tools, companies can immediately identify problems with triggered predictions requiring attention before they play into major business losses.
In addition, by utilising Cloud-to-Edge MLOps users can optimise data processing time because the models are preloaded and can process data faster than traditional methods like Hadoop or Spark would take. The shorter response time makes it possible for applications that need near real time output insights received during their usage cycle such as financial management applications or autonomous vehicle navigation systems both of which require quick response rates from queries sent within seconds or less from end points that are receiving live sensor input streams. Lastly, cost savings can be realised since storage capacity loadings per device will be reduced since only necessary external datasets need to be fetched for query processing across multiple points without having large datasets stored locally on these end point devices reducing storage costs over time for customers using these edge computing capabilities for their product offerings.
Automation and Streamlining of MLOps
The automation and streamlining of Machine Learning operations (MLOps) is seen as essential for the future of artificial intelligence (AI) and machine learning (ML). MLOps enables organisations to rapidly build, deploy and manage machine learning models into production, using various tools for model execution, analysis, and management. By streamlining the development process for models, MLOps provides businesses with a faster time-to-market compared to manual processes. As an organisation adopts an MLOps approach, they become more agile in their model development approach. This reduces time spent on engineering tasks such as model building or feature engineering, allowing them to better focus their data science teams on understanding business challenges and predicting solutions more aligned with customer needs.
Moreover, with the fast pace of change in AI/ML technologies, businesses need to quickly respond to changes in data sources — adding new features or updating existing ones — to stay competitive. With an automated process like MLOps, they can obtain real-time insights into their models’ performance while responding more quickly to both customer needs and market feedback. As models evolve and improve over time they will increasingly support automated decisions by end users but only if quality assurance measures such as system monitoring are present to ensure no degradation of outcomes.
Finally, it is also essential that organisations employ open source solutions whenever possible due to the maturity these solutions typically have across a wide range of use cases; this helps maximise reuse across multiple departments by ensuring that resources aren’t wasted on reinventing existing workflows or tools each time a project begins.
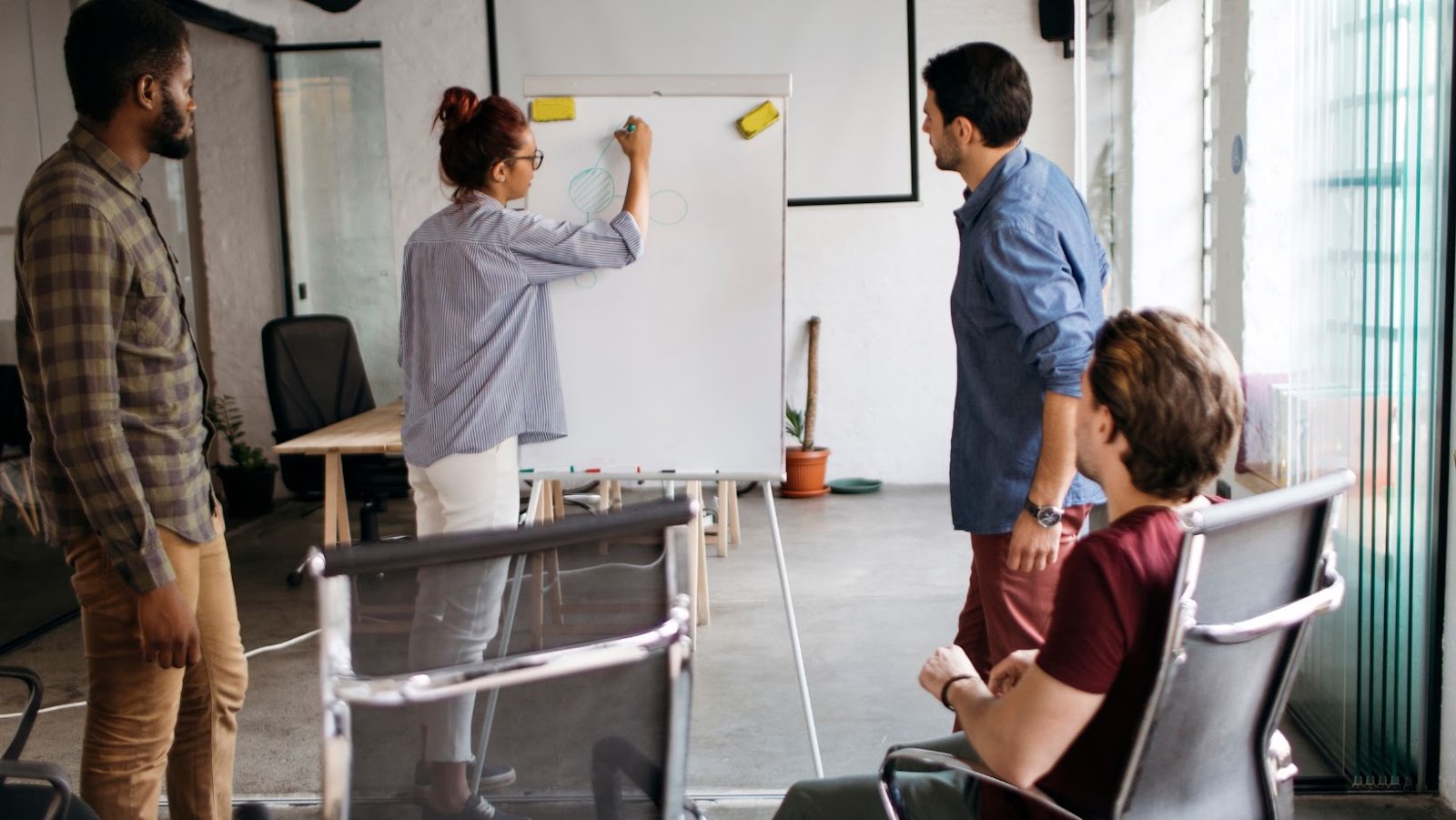
AI-driven MLOps
MLOps, or machine learning operations, is a set of practices intended to combine people, processes, and tools to enable collaboration and improve the machine learning lifecycle. While approaches to much of what is included in MLOps are traditional software engineering concepts, such as model training automation and unit testing, new technologies such as AI/ML-driven MLOps are significantly improving in this growing field.
AI-driven MLOps focuses on automation and integration with the existing AI/ML workflow through modern architectures such as containers, virtual machines and serverless. This helps teams reduce the manual steps typically required for development by leveraging existing infrastructure integrated into the organisations’ systems. Additionally, these configurations can be quickly updated or used for different projects, significantly reducing enterprise deployment times.
AI-driven MLOps also reduces risks associated with potential errors often seen in traditional software operations since these automated processes resemble how independent pieces communicate more closely than manual operations. This increased communication between components improves accuracy while detecting system trends quicker than a human can manually identify them.
Introducing AI-driven MLOps into an organisation’s pipeline enables them to move faster while also saving time and money – allowing businesses to quickly benefit from one or many projects without having to deploy resources or workloads into production until they are ready. Additionally, as organisations begin to take up this technology more broadly over time, they will continue to better understand data patterns while freeing up their development team’s capacity – enabling them to redeploy resources consistently across projects throughout their organisation.
Impact of Wallaroo Labs’ Investment
Wallaroo Labs’ $25M Series A round of funding from Microsoft’s M12 to help with MLOps from cloud-to-edge has major implications.
This investment will help accelerate the development and deployment of MLOps, allowing organisations to more easily set up and manage ML-powered applications at scale. It will also improve automation and efficiency, allowing businesses to gain insights and insight-driven actions.
Let’s explore the impact of Wallaroo Labs’ investment and what it means for the future of MLOps.
Increased Adoption of MLOps
The investment by Wallaroo Labs in MLOps signals a positive step forward in a relatively nascent but rapidly growing technology area. At its core, MLOps (or machine learning operations) is streamlining and automating the end-to-end production processes for developing machine learning models. Organisations can ensure repeatable, reliable and secure deployments for machine learning models by using DevOps best practices such as automation and version control.
This recent Wallaroo Labs announcement signals to the industry that MLOps is quickly becoming an important part of how companies are deploying production-ready artificial intelligence solutions. As more organisations turn to AI to solve real-world problems, MLOps will become necessary for efficient implementation, deployment, management and governance of AI elements. As such, increased adoption of MLOps will help ensure smooth operation and good performance from machine learning models over time.
Further adoption of MLOps is likely to drive new product innovation in this sector – plug ‘n’ play workflows that can self-organise and emulate existing development processes while adding dynamism will be key components to simplify complicated setups. In addition, improved operational agility among orgs as they learn to leverage these self-service resources will allow them greater flexibility with different system configurations while reducing cost overruns due to bad design choices or outright errors in the deployment cycle. These benefits should result in successful launch (and maintenance) of secure enterprise level production grade AI applications across industries worldwide.
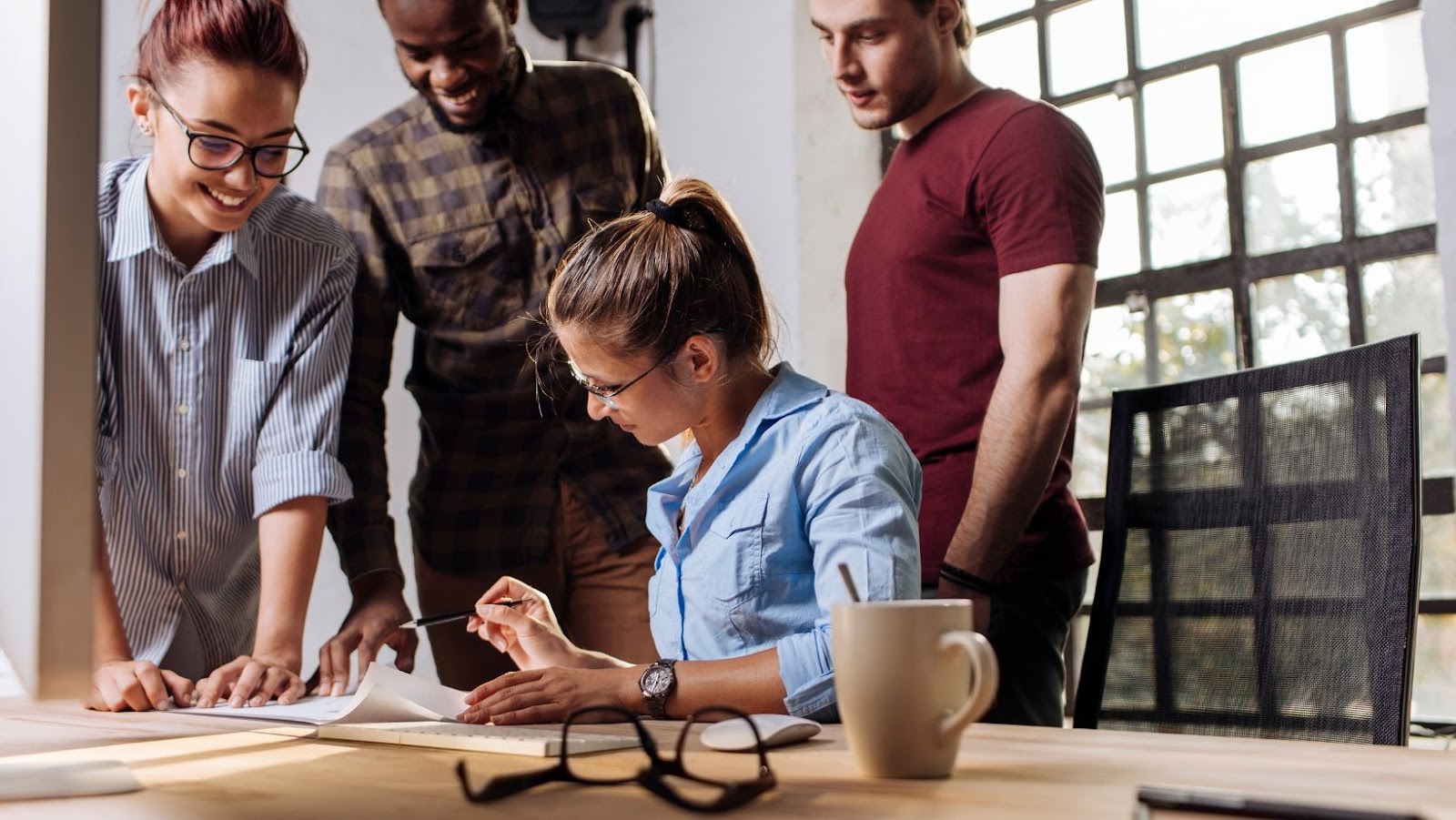
Increased Focus on MLOps
Wallaroo Labs, a distributed streaming platform provider, recently invested in MLOps. MLOps (machine learning operations) is a term used to refer to operationalization of machine learning models. This investment represents an increasing focus on making the development and deployment of complex AI models efficient and effective.
The Wallaroo Labs investment highlights the importance of MLOps in streamlining the integration of ML models into enterprise solutions. It signals an industry-wide recognition that organisations need automation and repeatability to get their data science projects into production faster and with more quality than in the past. They also understand that this can only be achieved if the machine learning lifecycle processes (from development, deployment, and testing to monitoring) are automated and linked.
With this additional support from Wallaroo Labs, we can expect to see improved tools for model management, tracking experiments, automated deployment pipelines for AI/ML systems, and easier ways for teams working on these systems to collaborate on various tasks with unified views across projects. This increased specialised focus on MLOps is critical for avoiding delays caused by manual processes when building production grade AI driven solutions. By investing further resources into this area of research and development, we are certain that there will be even more successful implementations of production ready AI throughout multiple industries in 2021 and beyond.
Improved MLOps Tools and Technologies
Wallaroo Labs recently announced an investment of $34 million in funding to continue advancing their MLOps (machine learning operations) tools and technologies. This significant contribution will help them to develop even more effective solutions that make it faster and easier to operationalize ML models at scale.
This news is incredibly exciting, as it will open up new opportunities for organisations interested in taking advantage of machine learning and artificial intelligence, while eliminating some of the biggest challenges they’ve faced when trying to do so. With improved MLOps tools, businesses can easily achieve rapid time-to-market with their models, gain greater accuracy across datasets and better manage costs associated with the project. This could lead to many powerful applications such as online retailing, optimization of customer service.
The investment also provides Wallaroo Labs with the resources necessary to continue serving as a leader in MLOps tools and technology development, allowing them to further democratise access to AI for businesses worldwide. Through this funding, teams can leverage Wallaroo Labs’ technology stack – including the Wallaroo Platform – to quickly deploy and manage machine learning applications across cloud architectures with maximum cost efficiency.
Overall, the future of MLOps looks bright thanks to Wallaroo Labs’ investment in this revolutionary space – exciting times indeed!